AI-based computational pathology. Using modern machine learning, interpretation of digital images of histopathology samples can be done with accuracy matching that of human experts – even superhuman performance has been achieved in some application areas. The AI-enabled computational pathology has the potential to lead to decision support tools helping pathologists work more efficiently, less subjectively, leading to harmonization of diagnoses and eventually, to improved care for the patients.
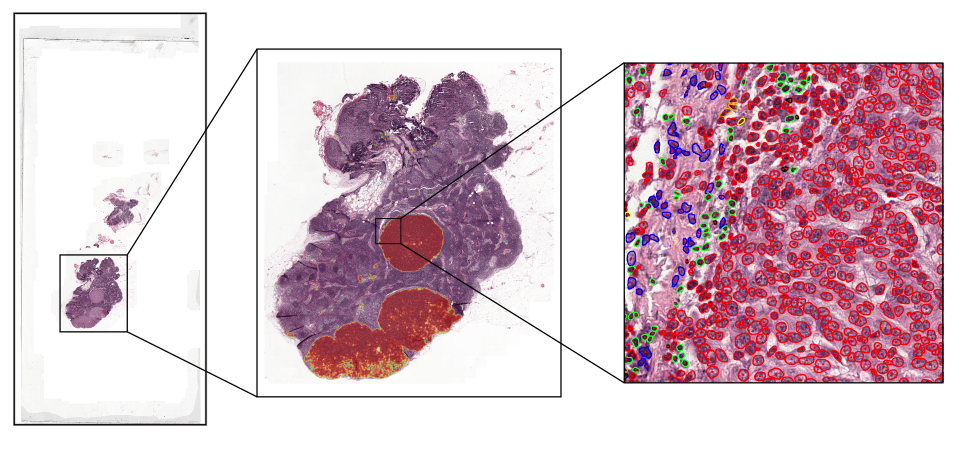
Label-free histology and microscopy: Generative AI-based virtual staining. Chemical staining makes the structure and morphology of cells and tissues visible, enabling visual interpretation and quantification of the structures in various applications. For example, H&E staining of tissue structures is the cornerstone in routine histopathology. Generative neural networks enable highly accurate image-to-image transforms, and this AI-based approach can be used for converting the unstained, label-free images of tissues into computationally virtual stained images, facilitating chemical-free tissue interpretation in various applications.
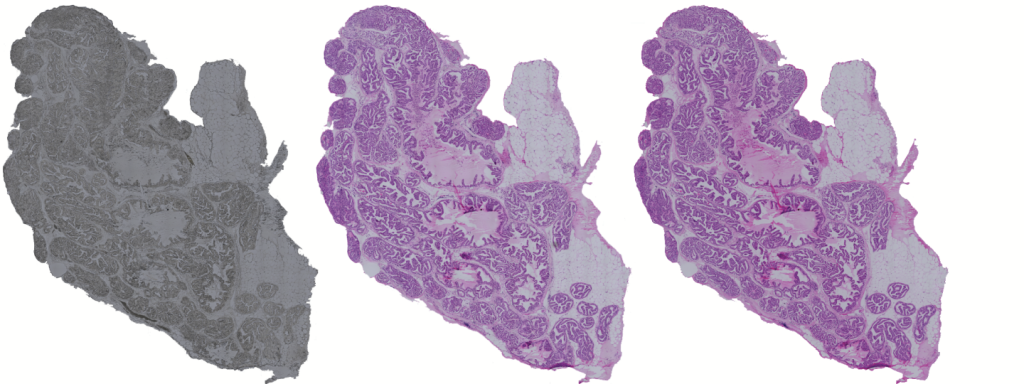
High-performance computing for scalable analysis of massive histopathology datasets. Modern deep learning based AI methods are data-hungry, with more training data typically leading to better accuracy and generalization performance. As a consequence, training of the methods requires massive computational power and data storage capacity. We pioneer in creating scalable AI-systems leveraging the massive digital pathology datasets and supercomputing. Our ComPatAI project will make use of the resources of LUMI supercomputer for the benefit of predictive histopathology.
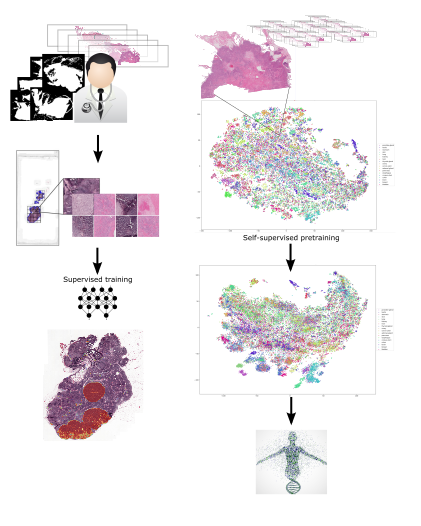
3D reconstruction and visualization of histology. Digital pathology typically refers to interpretation of histology from individual 2D sections of tissue. The original spatial appearance, however, is highly heterogeneous and three-dimensional. To enable visualization and quantitative analysis of tissue structures in their original 3D spatial context, we develop computational methods for whole slide image registration (alignment), 3D reconstruction, and 3D visualization. For advanced, immersive visualization and explorative interpretation of the 3D image stacks, we have pioneered in developing virtual reality (VR) applications for 3D histology.